-
Notifications
You must be signed in to change notification settings - Fork 43
New issue
Have a question about this project? Sign up for a free GitHub account to open an issue and contact its maintainers and the community.
By clicking “Sign up for GitHub”, you agree to our terms of service and privacy statement. We’ll occasionally send you account related emails.
Already on GitHub? Sign in to your account
VRPY column generation for a branch-bound-and-price algorithm #124
Comments
I am also using Best_Edges1, and remove_edges1 with alpha in [0.3, 0.5, 0.7, 0.9] |
Hi there ! This looks interesting. Please consider contributing to vrpy with your branch-and-bound framework and/or your modified greedy algorithm :) I am not suprised that the initialization method leads to different objective function values (with integer variables). I guess different columns can have the same relaxed objective function value, but different integer objective function values once the variables are integer. On the other hand, if you are referring to objective function values for the relaxed problem, then I am a bit lost to be honest. I am not sure why this is happening in your branch and bound tree. This is odd indeed. Perhaps we should first get the theory right, and then figure out where the implementation fails. A good place to ask such theoretical questions is over here. |
Hi, I will definitely contribute to vrpy/cspy for my work! And thank you for the link, I will ask around there. And yes, I meant that different initialization results in different values for the objective function of the relaxed problem, not the integer problem. I wonder - I defined my own forward labeling algorithm with many more resources. Thank you!! |
Hi @soomin-woo! edit Please link the question on OR-SO if you do ask, might get some super cool insight from the masters (Ruslan Sadykov et al.) Fleshed out some RCSP logic in torressa/cspy#98 |
Just had another thought. As the weight for each edge is being altered on the fly (dependant on resources), you may get a route which the final cost is falsely positive (or negative), which means that your linear relaxion is not (may not) being solved to optimality. The weights of each edge is set using the dual formulation (see pricing problem). |
@torressa, I'm sorry I took a while to answer. I was absorbing a lot of information everywhere, thanks to you! |
Just for reference, the question has been asked on OR-SE |
Hi @torressa, that question was me 👍 :) |
Hi @torressa, I found that the bug was in the pricing problem.
Currently, I am looking at the root node to figure out why the the negative column found after branching was not found at the root. I am trying to trace the bug to the exact place in the cspy but it's a bit difficult as the cspy lives in C++. We talked before that maybe it's because the edge cost is a function of the resource state at the last label. I know this is a lot to unpack, but I'd love to hear your opinion. Thank you as always! Soomin |
Hi again @soomin-woo! Thanks for checking this. |
Hi @torressa!
I am Soomin, and have asked you a few questions already in the past and I really appreciate what you have done so far.
I have applied cspy and vrpy to develop a branch-bound-and-price algorithm for our custom vehicle routing problem with electric vehicles.
I know branch and bound is not yet implemented by you but I wish to ask you some questions still, if that's okay. Your feedback will be very valuable to me!
What I have so far: The current column generation ends after running heuristic solvers of your choice, then the exact labelling algorithm (cspy), which finds no routes found with a negative reduced cost. Currently, I am only using forward direction as some features of my problem make it difficult to model the backward direction. Also, I have modified the cspy so that the edge weight is calculated dynamically as a function of the current resource state at the edge's starting node.
Current issue: When I do the branch and bound on the edge flows (for example, parent node: no restriction on the edge flow, left child branch: edge flow of (source, 1) >= 1, right child branch: edge flow of (source, 1) = 0), I sometimes get odd results. The output of the column generation result is sometimes better with the bounding constraints than the result without the constraints (for example, the parent node is worse than the child node). Note, this is considering the column generation results with linear relaxation.
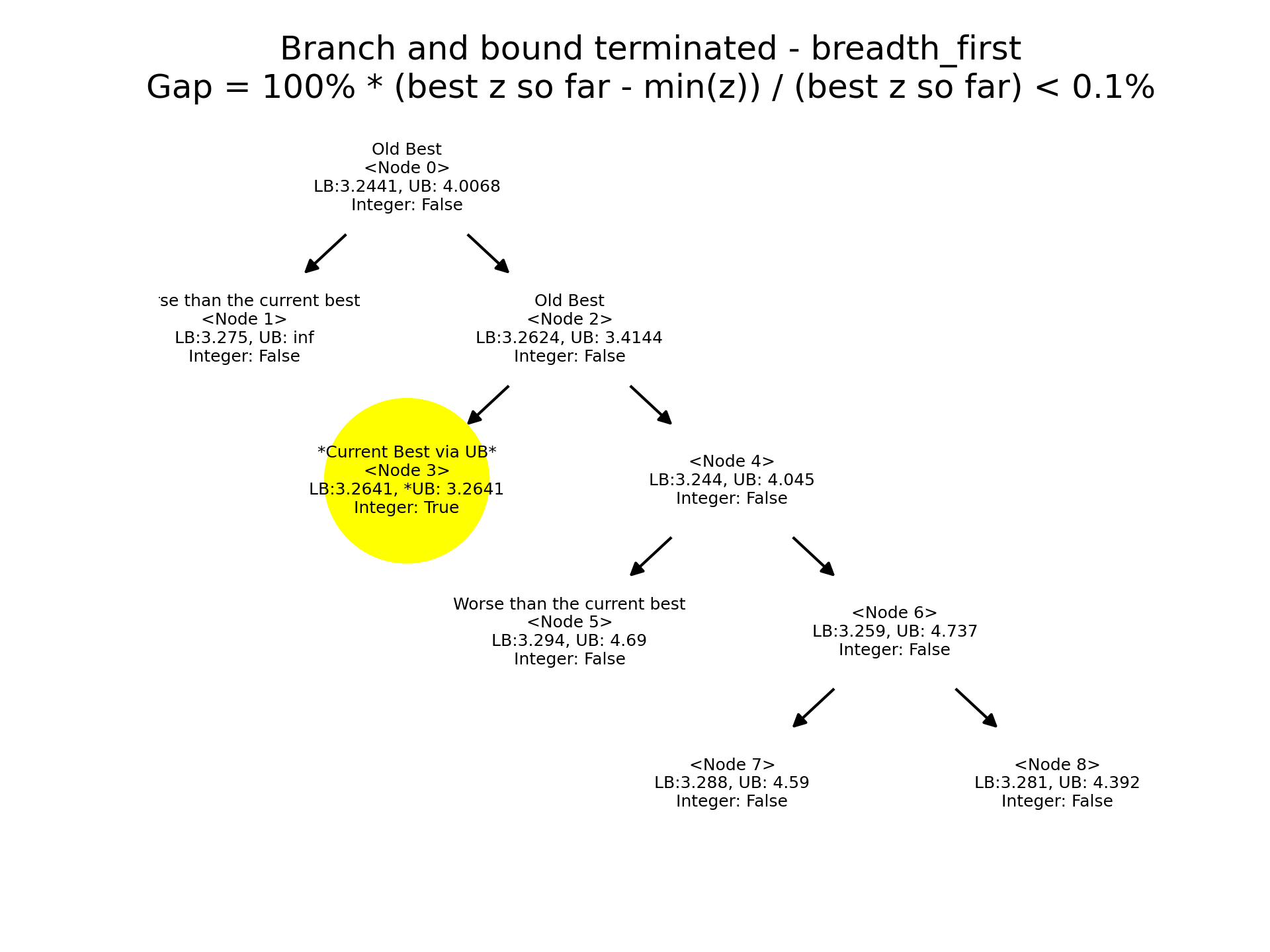
In the image attached, node 4 has the LB:3.244, which is the column generation result with linear relaxation and UB:4.045, which is the column generation result without linear relaxation. However, node 2 (parent of node 4) has the LB:3.2624 > 3.244, which is actually worse than node 4.
This means adding more constraints to the RMP actually improved the RMP's objective function value. It makes me question if I am solving the column generation to optimality, which is weird because the labeling algorithm via cspy must be exact. I am currently solving the subproblems using the cspy forward direction without any other heuristics and I am really stuck on this issue. Are there any parameters in the cspy/vrpy that I must pay attention to, which may determine the criteria for the optimality or the termination of CG? I have checked the threshold to determine if the reduced costs are indeed negative. I also checked that it's not a time-out or max iteration reached. They don't seem too problematic for me.
In addition, I noticed that different initialization methods actually result in different objective function values, not just the computation time - do you know anything about this? I implemented a modified version of the greedy algorithm to add an extensive amount of initial routes.
I'd really appreciate your feedback. Thank you so much!
-Soomin
The text was updated successfully, but these errors were encountered: