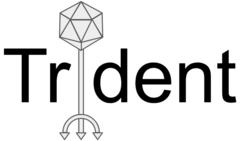
diff --git a/docs/404.html b/docs/404.html deleted file mode 100644 index d63247d..0000000 --- a/docs/404.html +++ /dev/null @@ -1,87 +0,0 @@ - - -
- - - - -vignettes/TrIdent-vignette.Rmd
- TrIdent-vignette.Rmd
TrIdent- Transduction -Identification
-TrIdent consists of three main functions which should be run in the -following order:
-TrIdentClassifier()
: Classifies
-contigs as ‘Prophage-like’, ‘Sloping’, ‘HighCovNoPattern’, and
-‘NoPattern’ using read coverage pattern-matching.plotTrIdentResults()
: Plots
-pattern-matching results of TrIdentClassifier()
.specializedTransductionID()
: Searches
-contigs classified as Prophage-like by TrIdentClassifier()
-for associated specialized transduction events.TrIdent automates the analysis of transductomics data by detecting, -classifying, and characterizing read coverage patterns associated with -potential transduction events. Transductomics, developed by Kleiner et -al. (2020), is a DNA sequencing-based method for the detection and -characterization of transduction events in pure cultures and complex -communities. Transductomics relies on mapping sequencing reads from a -viral-like particle (VLP)-fraction of a sample to contigs assembled from -the metagenome (whole-community) of the same sample. Reads from -bacterial DNA carried by VLPs will map back to the bacterial contigs of -origin creating read coverage patterns indicative of ongoing -transduction. The read coverage patterns detected represent DNA -being actively carried or transduced by VLPs. The read -coverage patterns do not represent complete -transduction events (i.e integration of transduced DNA -into new bacterial chromosomes).
-Reference: Kleiner, M., Bushnell, B., Sanderson, -K.E. et al. Transductomics: sequencing-based detection and analysis of -transduced DNA in pure cultures and microbial communities. Microbiome 8, -158 (2020). https://doi.org/10.1186/s40168-020-00935-5
- - -
-if (!require("pak", quietly = TRUE)) {
- install.packages("pak")
-}
-
-pak::pak("jlmaier12/TrIdent")
-library(TrIdent)
-if (!require("devtools", quietly = TRUE)) {
- install.packages("devtools")
-}
-
-devtools::install_github("jlmaier12/TrIdent")
-library(TrIdent)
Transductomics allows for the identification of bacterial DNA being -actively carried or transduced by VLPs. A transductomics dataset -consists of two parts- metagenomes from the whole-community and VLP -fractions of a sample. The whole-community fraction is generated by -extracting and sequencing DNA from the whole sample. The VLP-fraction is -generated by extraction and sequencing DNA of the ultra-purified VLPs in -the sample. VLP ultra-purification is generally done using CsCl -density-gradient ultracentrifugation. Additionally, it is very important -that the VLP-fraction is treated with DNase to remove free DNA! After -sequencing, reads from the whole-community fraction are assembled and -both the whole-community and VLP-fraction reads are mapped to the -assembly. Read mapping should be performed -using a high minimum identity (0.97 or higher) and -random mapping of ambiguous reads. The pileup files needed for -TrIdent are generated using the .bam files produced during read -mapping.
-Deep sequencing of the whole-community and VLP-fractions is -needed for transductomics! Sample preparation, -sequencing procedures, and bioinformatics methods are -detailed in Kleiner -et al.(2020).
-TrIdent detects read coverage patterns using a pattern-matching -algorithm that operates on pileup files. A pileup file is a file format -where each row summarizes the ‘pileup’ of reads at specific genomic -locations. Pileup files can be used to generate a rolling mean of read -coverages and associated base pair positions across a metagenome -assembly which reduces data size while preserving read coverage -patterns. TrIdent requires that input pileups files be generated -using a 100 bp window/bin size.
-Some read mappers, like BBMap,
-will allow for the generation of pileup files in the bbmap.sh
-command with the use of the bincov
output with the
-covbinsize=100
parameter/argument. Otherwise,
-BBMap’s pileup.sh
-can convert .bam files produced by any read mapper to pileup
-files compatible with TrIdent using the
-bincov
output with binsize=100
.
TrIdent requires two pileup files from a transductomics dataset as -input:
-Remember- The data used for each pileup file must originate -from the same sample. Pileup files must use a 100 bp -window/bin size for the rolling mean.
-The input pileup files must have the following -format:
-Dataframe with four columns:
--V1 - | --V2 - | --V3 - | --V4 - | -
---|---|---|---|
-NODE_62 length_245921_cov_3.25857_ID_9556347 - | --0 - | --100 - | --20626396 - | -
-NODE_62 length_245921_cov_3.25857_ID_9556347 - | --0 - | --200 - | --20626496 - | -
-NODE_62 length_245921_cov_3.25857_ID_9556347 - | --0 - | --300 - | --20626596 - | -
-NODE_62 length_245921_cov_3.25857_ID_9556347 - | --0 - | --400 - | --20626696 - | -
-NODE_62 length_245921_cov_3.25857_ID_9556347 - | --0 - | --500 - | --20626796 - | -
-NODE_62 length_245921_cov_3.25857_ID_9556347 - | --0 - | --600 - | --20626896 - | -
TrIdentClassifier()
is the main function in TrIdent.
-This function filters contigs based on length and read coverage,
-performs pattern-matching to classify contigs, identifies highly
-active/abundant and heterogenously integrated Prophage-like elements,
-determines which contigs have high VLP-fraction:whole-community read
-coverage ratios, identifies start and stop positions and sizes of
-pattern-matches, and calculates slopes for Sloping pattern-matches.
Note that TrIdentClassifier()
results should not be
-taken at face value and some manual curation is needed! We recommend
-careful examination of the output plots to determine if you agree with
-the TrIdentClassifier()
classifications.
Contigs that are too short or have little to no read coverage are
-filtered out prior to pattern-matching. TrIdentClassifier()
-filters out contigs that do not have at least 10x coverage on a total of
-5,000 bp across the whole contig. The read coverage filtering was done
-in this way to avoid filtering out long contigs with short Prophage-like
-patterns that might get removed if filtering was done with read coverage
-averages or medians. Additionally, contigs less than 30,000 bp are
-filtered out by default, however this can be changed with the
-minContigLength
parameter. While contigs shorter than
-30,000 bp may be poor quality and are often not long enough to capture
-complete transduction patterns, we allow users to set a minimum
-minContigLength
of 25,000 bp. If you would like to
-reduce the size of your input pileup files for TrIdent,
-consider pre-filtering your assembly for contigs greater than
-25,000 bp prior to read mapping!
It is expected that the majority of contigs in the dataset -are filtered out due to low read coverage during this -step! If very few contigs are filtered out due to low -read coverage, this may be an indication of DNA -contamination in your VLP-fraction.
-The input pileup files have 100 bp windows in which the mapped read
-coverage is averaged over. This small window size is needed to detect
-read coverage patterns associated with specialized transduction which
-may only span a few thousand basepairs. However, read coverage patterns
-associated with other types of transduction generally don’t require the
-resolution that 100 bp windows provide. While users can use the 100 bp
-windowSize
for TrIdentClassifier()
, the
-processing time will be increased significantly and noisy data may
-interfere with pattern-matching. We find that the default 1,000 bp
-windowSize
provides a nice balance between processing time
-and read coverage pattern resolution.
TrIdentClassifier()
detects read coverage patterns in
-the VLP-fraction using a pattern-matching approach. Several predefined
-patterns, described below, are built using the specific length and read
-coverage values of the contig being assessed. Patterns are translated
-across each contig in 1,000 bp sliding windows and at each translation,
-a pattern-match score is calculated by taking the mean absolute
-difference of the VLP-fraction read coverage and the pattern values. The
-smaller the match-score, the better the pattern-match. After a pattern
-is fully translated across a contig, certain aspects of the pattern are
-changed (i.e. height, width, slope) and translation is repeated. This
-process of translation and pattern re-scaling is repeated until a large
-number of pattern variations are tested. After pattern-matching is
-complete, the pattern associated with the best match-score is used for
-contig classification. Contigs are classified as ‘Prophage-like’,
-‘Sloping’, or ‘NoPattern’ during pattern-matching.
There are four sloping pattern variations in the sloping pattern
-class. The sloping patterns are representative of large DNA transfers
-that take place during generalized, lateral and gene transfer agent
-(GTA) transduction due to the decreasing frequency of DNA packaging
-moving away from the packaging initiation sites. During
-pattern-matching, the slope values of the sloping patterns are decreased
-until a minimum slope of 0.001 (change of 10x read coverage over 10,000
-bp) is reached. The minimum slope value can be changed with the
-minSlope
parameter. Generalized, lateral and GTA
-transduction events can span tens to hundreds of kilobasepairs of DNA
-and a single contig typically does not capture an entire event.
-Depending on which part of the transducing event is captured by the
-contig, the sloping can be very severe or almost 0. Patterns 1 and 2
-represent contigs that capture a Sloping transducing event somewhere in
-the middle of the DNA transfer. Patterns 2 and 4 represent contigs that
-capture the jump of read coverage associated with packaging initiation
-site of a Sloping transducing event. Patterns 2 and 4 are translated
-across the contig in addition to having the slopes changed while only
-the slopes are changed on patterns 1 and 2.
There are three block patterns in the Prophage-like pattern class.
-The block patterns are representative of integrated genetic elements
-that can be excised from the host chromosome and mobilized. The blocks
-of read coverage that define this classification are formed when the
-reads of mobilized genetic elements packaged by VLPs map back to their
-respective integration sites in the host bacterium’s chromosome.
-Prophage, phage-inducible chromosomal islands (PICIs), and transposons
-are all examples of genetic elements that fall into the Prophage-like
-class. During pattern-matching, both the heights and widths of block
-patterns are altered and all pattern variations are translated across
-the contig. The block pattern widths never get smaller than 10,000 bp by
-default, however this can be changed with the minBlockSize
-parameter. Pattern 1 represents a Prophage-like element that is entirely
-on the contig while patterns 2 and 3 represent Prophage-like elements
-that trail off the right or left side of the contig, respectively. While
-a Prophage-like classification is not an example of transduction by
-itself, there may be transduction associated with Prophage-like
-classifications. The improper excision of Prophage-like elements may
-lead to the specialized transduction of genes neighboring the element’s
-integration site. TrIdent’s specializedTransductionID()
-function (explained in detail below) will attempt to detect specialized
-transduction events associated with Prophage-like classifications.
Since the best pattern-match for each contig is determined by -comparing match-scores amongst all pattern-variations from all pattern -classes, we needed a ‘negative control’ pattern to compare against. The -‘NoPattern’ ‘pattern’ serves as a negative control by matching to -contigs with no read coverage patterns. We made two NoPattern patterns -which consist of a horizontal line the same length as the contig being -assessed at either the average or median read coverage for a contig. -This pattern is not re-scaled or translated in any way. Note that read -coverage patterns are heavily dependent on the depth of read coverage -achieved during sequencing and therefore very rare transduction events -may not achieve sufficient read coverage for detection with read -coverage pattern-matching. Rather than label contigs with no read -coverage pattern as having ‘no transduction’, we instead label them as -having ‘no pattern’.
-Prophage-like elements that are actively replicating or are highly
-abundant will typically generate more sequencing reads than the rest of
-their host bacterium’s chromosome. This may create a region of elevated
-read coverage at the element’s insertion site in the whole-community
-fraction read coverage. Conversely, if a Prophage-like element is
-integrated into only a portion of the host bacterial population, there
-may be a dip or depression in read coverage at the integration site in
-the whole-community read coverage. In order to determine if the
-whole-community read coverage is elevated or depressed at the site of a
-Prophage-like element, one must know the Prophage-like element’s genomic
-location. While some tools rely on annotation information to identify
-Prophage-like elements in whole-community metagenomes,
-TrIdentClassifier()
uses the VLP-fraction read coverage
-patterns. The locations of Prophage-like pattern-matches are used to
-calculate the Prophage-like:non-Prophage-like whole-community read
-coverage ratio. Prophage-like patterns with whole-community read
-coverage ratios greater than 1.15 are labeled as ‘elevated’ while ratios
-less then 0.75 are labeled as ‘depressed’.
If a contig receives a noPattern classification, it proceeds to an -additional classification step which either leaves the classification as -is or re-classifies the contig as having a high -VLP-fraction:whole-community read coverage ratio (‘HighCovNoPattern’). -In other words, contigs with the HighCovNoPattern classification have -even read coverage across the contig (i.e. no notable read coverage -pattern) and the VLP-fraction read coverage is notably higher than the -whole-community read coverage. This re-classification is necessary -because certain biologically significant events that may be of interest -to the user can have these characteristics. First, certain transduction -events, specifically those associated with the Sloping classification, -may be so long that they extend across multiple contigs. The ‘tails’ of -the sloping patterns created by these events may have little to no -actual sloping (no pattern) but relatively high read coverage (Fig S2 -Kleiner et al., 2020). Second, there is evidence that membrane vesicle -(MV) mediated transduction (also known as vesiduction or protected -extracellular transformation) produces relatively even read coverage -patterns when purified MV sequencing reads are mapped back to their -bacterial chromosome of origin (Faddetta et al., 2022). Lastly, phage -genomes that have assembled into contigs in the whole-community fraction -may generate high levels of even read coverage as the phage reads in the -VLP-fraction map back to their own genome sequences. Contigs with median -VLP-fraction:Whole-community read coverage ratios greater than 2, in -other words contigs where the median VLP-fraction read coverage value is -2x the whole-community median read coverage value, are re-classified as -HighCovNoPattern.
-Reference: Faddetta, T., Vassallo, A., Del Duca, S. -et al. Unravelling the DNA sequences carried by Streptomyces coelicolor -membrane vesicles. Sci Rep 12, 16651 (2022). https://doi.org/10.1038/s41598-022-21002-z
-Default arguments:
-
-TrIdentOutput <- TrIdentClassifier(
- VLPpileup = VLPFractionSamplePileup,
- WCpileup = WholeCommunitySamplePileup
-)
-#> Reformatting pileup files
-#> Starting pattern-matching...
-#> A quarter of the way done with pattern-matching
-#> Half of the way done with pattern-matching
-#> Almost done with pattern-matching!
-#> Determining sizes (bp) of pattern matches
-#> Identifying highly active/abundant or heterogenously integrated
-#> Prophage-like elements
-#> Finalizing output
-#> Execution time: 23.19secs
-#> 1 contigs were filtered out based on low read coverage
-#> 0 contigs were filtered out based on length
-#>
-#> HighCovNoPattern NoPattern Prophage-like Sloping
-#> 1 1 4 3
-#> 3 of the prophage-like classifications are highly active or abundant
-#> 1 of the prophage-like classifications are mixed, i.e. heterogenously
-#> integrated into their bacterial host population
-TrIdentClassifier(VLPpileup, WCpileup,
- windowSize = 1000, minBlockSize = 10000,
- maxBlockSize = Inf, minContigLength = 30000, minSlope = 0.001,
- suggFiltThresh = FALSE, SaveFilesTo
-)
VLPpileup
: VLP-fraction pileup file.WCpileup
: Whole-community pileup file.windowSize
: The number of basepairs to average read
-coverage values over. Options are 100, 200, 500, 1000 ONLY. Default is
-1000.minBlockSize
: The minimum size (in bp) of the
-Prophage-like block pattern. Default is 10000. Must be greater than
-1000.maxBlockSize
: The maximum size (in bp) of the
-Prophage-like block pattern. Default is NA (no maximum).minContigLength
: The minimum contig size (in bp) to
-perform pattern-matching on. Must be at least 25000. Default is
-30000.minSlope
: The minimum slope value to test for sloping
-patterns. Default is 0.001 (i.e minimum change of 10x read coverage over
-100,000 bp).suggFiltThresh
: TRUE or FALSE, Suggest a filtering
-threshold for TrIdent classifications based on the normalized
-pattern-match scores. Default is FALSE.SaveFilesTo
: Optional, Provide a path to the directory
-you wish to save output to. A folder will be made within the provided
-directory to store results.TrIdentClassifier()
outputs a histogram displaying the
-overall abundance and quality of pattern-matches in addition to the
-composition of classifications. The displayed pattern-match scores are
-normalized by dividing each score by its associated contig length. The
-scores are normalized to visualize the overall quality of
-pattern-matching for the entire dataset. Remember, smaller pattern-match
-scores correspond to better pattern-matches.
The output of TrIdentClassifier()
is a list containing
-five objects:
windowSize
used.Save the desired list-item to a new variable using its associated -name.
-Summary table:
-
-TrIdentSummaryTable <- TrIdentOutput$SummaryTable
-contigName - | --classifications - | --normMatchScore - | --VLPWCRatio - | --matchSize - | --startPosBp - | --endPosBp - | --proLikeWCReadCov - | --proLikeWCReadCovRatio - | --slope - | -
---|---|---|---|---|---|---|---|---|---|
-NODE_62 - | --Prophage-like - | --0.1428571 - | --NA - | --171000 - | --62000 - | --233000 - | --Elevated - | --1.4880 - | --NA - | -
-NODE_135 - | --Prophage-like - | --0.2737766 - | --NA - | --32000 - | --149000 - | --181000 - | --Elevated - | --1.2795 - | --NA - | -
-NODE_1088 - | --Sloping - | --0.0802549 - | --NA - | --63000 - | --1000 - | --64000 - | --NA - | --NA - | --0.0024 - | -
-NODE_352 - | --Sloping - | --0.1829770 - | --NA - | --121000 - | --1000 - | --122000 - | --NA - | --NA - | ---0.0001 - | -
-NODE_368 - | --Prophage-like - | --0.1530534 - | --NA - | --30000 - | --26000 - | --56000 - | --Depressed - | --0.4001 - | --NA - | -
-NODE_560 - | --HighCovNoPattern - | --0.0694395 - | --16.6016 - | --95000 - | --1000 - | --96000 - | --NA - | --NA - | --NA - | -
-NODE_617 - | --Prophage-like - | --0.1613141 - | --NA - | --48000 - | --34000 - | --82000 - | --Elevated - | --1.7726 - | --NA - | -
-NODE_1401 - | --NoPattern - | --0.1006696 - | --0.0192 - | --54000 - | --1000 - | --55000 - | --NA - | --NA - | --NA - | -
-NODE_2060 - | --Sloping - | --0.1037661 - | --NA - | --27000 - | --1000 - | --28000 - | --NA - | --NA - | --0.0275 - | -
plotTrIdentResults()
allows users to visualize both the
-whole-community and VLP-fraction read coverage and the pattern-match
-associated with each contig classified as Prophage-like, Sloping and
-HighCovNoPattern.
The TrIdentClassifier()
output contains information
-needed to re-build each pattern-match used for contig classification. To
-re-build a complete pattern-match for visualization,
-plotTrIdentResults()
uses the pattern-match’s minimum and
-maximum values and the start and stop positions.
The whole-community and VLP-fraction read coverage are plotted for -each contig classified as Prophage-like, Sloping or HighCovNoPattern. -The pattern-match associated with the classification is overlaid on the -VLP-fraction read coverage. The whole-community read coverage is -displayed for visualization of elevated or depressed Prophage-like -region read coverage.
-Default arguments:
-
-TrIdentPlots <- plotTrIdentResults(
- VLPpileup = VLPFractionSamplePileup,
- WCpileup = WholeCommunitySamplePileup,
- TrIdentResults = TrIdentOutput
-)
-plotTrIdentResults(
- VLPpileup, WCpileup, TrIdentResults, matchScoreFilter,
- saveFilesTo
-)
VLPpileup
: VLP-fraction pileup file.WCpileup
: Whole-community pileup file.TrIdentResults
: The output from
-TrIdentClassifier()
-matchScoreFilter
: Optional, Filter plots using the
-normalized pattern match-scores. A suggested filtering threshold is
-provided by TrIdentClassifier()
if
-suggFiltThresh=TRUE
.saveFilesTo
: Optional, Provide a path to the directory
-you wish to save output to. A folder will be made within the provided
-directory to store results.The output of plotTrIdentResults()
is a list containing
-ggplot objects. The list contains all read coverage plots for contigs
-classified as Sloping, Prophage-like, or HighCovNoPattern and their
-respective pattern-matches.
By default, the plots are displayed with raw read coverage
-values. We recommend that users also view plots using
-logScale=TRUE
as some specialized transduction patterns
-occur at such low frequencies they can only be visualized using log
-scaled read coverage values.
View all plots:
-
-TrIdentPlots
View one plot:
-
-TrIdentPlots$NODE_62
Specialized transduction occurs when an integrated Prophage-like
-element both excises and packages a small portion of bacterial DNA
-directly outside the borders of its insertion site.
-specializedTransductionID()
searches contigs classified as
-Prophage-like for dense read coverage outside the borders of the
-Prophage-like patterns that might represent specialized transduction
-events. Because specialized transduction tends to be fairly short (a
-couple kbps) compared to generalized, lateral or GTA transduction (tens
-to hundreds of kbps), averaging read coverage over a 1,000 bp distance
-(i.e using a windowSize=1000
) can ‘blur’ specialized
-transduction patterns. This is why specialized transduction is not
-identified in TrIdentClassifier()
. Instead, we use the
-locations of Prophage-like elements identified with
-TrIdentClassifier()
to guide our search for specialized
-transduction in specializedTransductionID()
.
Note that specializedTransductionID()
results should not
-be taken at face value and some manual curation is needed! We recommend
-careful examination of the output plots to determine if you agree with
-the specializedTransductionID()
results. Setting
-logScale=TRUE
will plot the read coverage in log10 scale
-and may help with visualization of low-frequency specialized
-transduction events. Be aware that in some cases, log scaled read
-coverage can obfuscate the Prophage-like element pattern itself.
Since, specialized transduction read coverage patterns are relatively
-small, specializedTransductionID()
‘zooms-in’ on
-Prophage-like elements to aid with specialized transduction
-visualization. To ‘zoom-in’ on Prophage-like elements,
-specializedTransductionID()
uses the pattern-match
-information generated in TrIdentClassifier()
to subset the
-contig 50,000 bp outside the pattern-match borders.
Since the windowSize
used for pattern-matching in
-TrIdentClassifier()
is likely larger than 100 bp,
-the Prophage-like pattern-match border locations will not directly
-translate to the border locations at the 100 bp windowSize
.
-To re-identify the borders of Prophage-like elements, we use a rolling
-standard deviation (SD) to detect large changes in read coverage
-associated with Prophage-like element borders. First, the contig is
-subset surrounding the left and right pattern-match borders. For contigs
-that have a Prophage-like pattern-match that trails off the side of a
-contig, then only the border that falls on the contig is subset. Next,
-for each subset, the rolling SD is calculated between each read coverage
-value and the contig position associated with the largest SD is chosen
-as the border location. The border locations are marked on the output
-plots with black vertical lines.
Once the Prophage-like element borders are identified,
-specializedTransductionID()
starts from the border
-locations and searches outwards for dense read coverage that meet the
-‘requirements’ for specialized transduction as defined by two parameters
-in specializedTransductionID()
:
noReadCov
specTransLength
specializedTransductionID()
first makes sure that any
-coverage it detects outside the borders is not disrupted by a defined
-region of no read coverage (noReadCov
). The default value
-for noReadCov
is 500 bp. Secondly,
-specializedTransductionID
ensures that any read coverage it
-detects outside of the Prophage-like borders meets a minimum length
-requirement (specTransLength
). The default value for
-specTransLength
is 2000 bp. So by default,
-specializedTransductionID()
will search for coverage
-directly outside of the Prophage-like borders that is at least 2000 bp
-long and is not interrupted at any point by more than 500 bp of no read
-coverage. If these requirements are met,
-specializedTransductionID()
will label the contig as having
-specialized transduction. The ‘end’ of the specialized transduction
-region is marked on the output plots with red vertical lines.
-Additionally, read coverage plots for contigs with specialized
-transduction are colored green instead of blue.
Default arguments:
-
-SpecTransduc <- specializedTransductionID(
- VLPpileup = VLPFractionSamplePileup,
- TrIdentResults = TrIdentOutput
-)
-#> 2 contigs have potential specialized transduction
-#> We recommend that you also view the results of this search with
-#> logScale=TRUE
-specializedTransductionID(VLPpileup, TrIdentResults,
- noReadCov = 500, specTransLength = 2000,
- logScale = FALSE, matchScoreFilter,
- SaveFilesTo, specificContig
-)
VLPpileup
: VLP-fraction pileup file.TrIdentResults
: The output from
-TrIdentClassifier()
.noReadCov
: Number of basepairs of zero read coverage
-encountered before specialized transduction searching stops. Default is
-500. Must be at least 100.specTransLength
: Number of basepairs of non-zero read
-coverage needed for specialized transduction to be considered. Default
-is 2000. Must be at leastlogScale
: TRUE or FALSE, display VLP-fraction read
-coverage in log10 scale. Default is FALSE.matchScoreFilter
: Optional, Filter plots using the
-normalized pattern match-scores. A suggested filtering threshold is
-provided by TrIdentClassifier()
if
-suggFiltThresh=TRUE
.SaveFilesTo
: Optional, Provide a path to the directory
-you wish to save output to. A folder will be made within the provided
-directory to store results.specificContig
: Optional, Search a specific contig
-classified as Prophage-like (“NODE_1”).The output of specializedTransductionID()
is a list
-containing two objects:
specializedTransductionID()
results for all contigs
-classified as Prophage-like in TrIdentClassifier()
.specializedTransductionID()
-are marked on each plot with black vertical lines. If
-specializedTransductionID()
identifies potential
-specialized transduction, the plot will be colored green instead of
-blue. The end of specialized transduction will be marked with a red
-vertical line.Save the desired list-item to a new variable using its associated -name.
-Summary table:
-
-SpecializedTransducSummaryTable <- SpecTransduc$summaryTable
-contigName - | --specTransduc - | --left - | --right - | --lengthLeft - | --lengthRight - | -
---|---|---|---|---|---|
-NODE_62 - | --yes - | --yes - | --no - | --45400 - | --NA - | -
-NODE_135 - | --no - | --no - | --no - | --NA - | --NA - | -
-NODE_368 - | --no - | --no - | --no - | --NA - | --NA - | -
-NODE_617 - | --yes - | --yes - | --yes - | --33300 - | --9800 - | -
View all plots:
-
-SpecTransduc$Plots
View a specific plot:
-
-SpecTransduc$Plots$NODE_62
If you’d like to combine the summary tables produced by
-TrIdentClassifier()
and
-specializedTransductionID()
, try the following:
-FinalTrIdentSummaryTable <- merge(TrIdentSummaryTable,
- SpecializedTransducSummaryTable,
- by = "contigName", all.x = TRUE
-)
-contigName - | --classifications - | --normMatchScore - | --VLPWCRatio - | --matchSize - | --startPosBp - | --endPosBp - | --proLikeWCReadCov - | --proLikeWCReadCovRatio - | --slope - | --specTransduc - | --left - | --right - | --lengthLeft - | --lengthRight - | -
---|---|---|---|---|---|---|---|---|---|---|---|---|---|---|
-NODE_1088 - | --Sloping - | --0.0802549 - | --NA - | --63000 - | --1000 - | --64000 - | --NA - | --NA - | --0.0024 - | --NA - | --NA - | --NA - | --NA - | --NA - | -
-NODE_135 - | --Prophage-like - | --0.2737766 - | --NA - | --32000 - | --149000 - | --181000 - | --Elevated - | --1.2795 - | --NA - | --no - | --no - | --no - | --NA - | --NA - | -
-NODE_1401 - | --NoPattern - | --0.1006696 - | --0.0192 - | --54000 - | --1000 - | --55000 - | --NA - | --NA - | --NA - | --NA - | --NA - | --NA - | --NA - | --NA - | -
-NODE_2060 - | --Sloping - | --0.1037661 - | --NA - | --27000 - | --1000 - | --28000 - | --NA - | --NA - | --0.0275 - | --NA - | --NA - | --NA - | --NA - | --NA - | -
-NODE_352 - | --Sloping - | --0.1829770 - | --NA - | --121000 - | --1000 - | --122000 - | --NA - | --NA - | ---0.0001 - | --NA - | --NA - | --NA - | --NA - | --NA - | -
-NODE_368 - | --Prophage-like - | --0.1530534 - | --NA - | --30000 - | --26000 - | --56000 - | --Depressed - | --0.4001 - | --NA - | --no - | --no - | --no - | --NA - | --NA - | -
-NODE_560 - | --HighCovNoPattern - | --0.0694395 - | --16.6016 - | --95000 - | --1000 - | --96000 - | --NA - | --NA - | --NA - | --NA - | --NA - | --NA - | --NA - | --NA - | -
-NODE_617 - | --Prophage-like - | --0.1613141 - | --NA - | --48000 - | --34000 - | --82000 - | --Elevated - | --1.7726 - | --NA - | --yes - | --yes - | --yes - | --33300 - | --9800 - | -
-NODE_62 - | --Prophage-like - | --0.1428571 - | --NA - | --171000 - | --62000 - | --233000 - | --Elevated - | --1.4880 - | --NA - | --yes - | --yes - | --no - | --45400 - | --NA - | -
-sessionInfo()
-#> R version 4.2.1 (2022-06-23 ucrt)
-#> Platform: x86_64-w64-mingw32/x64 (64-bit)
-#> Running under: Windows 10 x64 (build 19045)
-#>
-#> Matrix products: default
-#>
-#> locale:
-#> [1] LC_COLLATE=English_United States.utf8
-#> [2] LC_CTYPE=English_United States.utf8
-#> [3] LC_MONETARY=English_United States.utf8
-#> [4] LC_NUMERIC=C
-#> [5] LC_TIME=English_United States.utf8
-#>
-#> attached base packages:
-#> [1] stats graphics grDevices utils datasets methods base
-#>
-#> other attached packages:
-#> [1] kableExtra_1.4.0 ggplot2_3.5.1 patchwork_1.3.0 knitr_1.49
-#> [5] TrIdent_0.0.0.9000
-#>
-#> loaded via a namespace (and not attached):
-#> [1] Rcpp_1.0.13-1 bslib_0.8.0 compiler_4.2.1 pillar_1.9.0
-#> [5] jquerylib_0.1.4 tools_4.2.1 digest_0.6.37 viridisLite_0.4.2
-#> [9] jsonlite_1.8.9 evaluate_1.0.1 lifecycle_1.0.4 tibble_3.2.1
-#> [13] gtable_0.3.6 pkgconfig_2.0.3 rlang_1.1.4 cli_3.6.2
-#> [17] rstudioapi_0.17.1 roll_1.1.7 yaml_2.3.10 pkgdown_2.1.1
-#> [21] xfun_0.49 fastmap_1.2.0 xml2_1.3.6 withr_3.0.2
-#> [25] stringr_1.5.1 dplyr_1.1.4 generics_0.1.3 desc_1.4.3
-#> [29] fs_1.6.5 vctrs_0.6.5 htmlwidgets_1.6.4 sass_0.4.9
-#> [33] systemfonts_1.1.0 grid_4.2.1 tidyselect_1.2.1 svglite_2.1.3
-#> [37] glue_1.8.0 R6_2.5.1 textshaping_0.4.1 fansi_1.0.6
-#> [41] rmarkdown_2.29 purrr_1.0.2 tidyr_1.3.1 farver_2.1.2
-#> [45] magrittr_2.0.3 scales_1.3.0 htmltools_0.5.8.1 colorspace_2.1-1
-#> [49] labeling_0.4.3 ragg_1.3.3 utf8_1.2.4 stringi_1.8.4
-#> [53] RcppParallel_5.1.9 munsell_0.5.1 cachem_1.1.0
TrIdent (Transduction Identification) is a reference-independent tool for the automated detection, classification and characterization of read coverage patterns in transductomics datasets.
-