Which RDM comparison metric to use? #325
Replies: 2 comments 3 replies
-
Hi Silvia, |
Beta Was this translation helpful? Give feedback.
-
Hi @silviaformica, as you have so few conditions, you probably have to predict the actual size of the distances, i.e. cannot use rank correlations effectively. As I don't think you do not necessarily want to predict that the 0 distances are actually 0, I think you want a correlation distance not a cosine like distance. Thus, I would suggest to use the Pearson correlation or its whitened version. The Pearson correlation effectively becomes a differences between the normalised 1 and 0 predicted distances in your case, which is easy to interpret. The whitened one is expected to yield slightly higher power, but is a more complicated computation. P.S.: a note on the linear weighting: This would mean that you take your three RDMs and combine them to produce a prediction for the RDM. For your use-case you would then probably interpret the weights for the different components. We provide a linear model in the toolbox that you could use for fitting this, but the fitted parameters do not come with error bars or tests at the moment. |
Beta Was this translation helpful? Give feedback.
-
Dear all,
I am fairly new to RSA and although I have been learning a lot lately thanks to the demos and tutorials of this toolbox, I am still lacking a good background in math and data analysis to make some crucial informed decisions about my study.
I have a EEG dataset, including two similar tasks. In each of the two tasks, experimental conditions are organized in a 2x2 factorial design (so in total I have 2: Task * 2: ConditionME * 2: ConditionYOU). I hypothesize some differences in how conditionME, conditionYOU and their interaction are represented across the two tasks.
This image shows the 3 RDM models that I hypothesize based on my experimental design
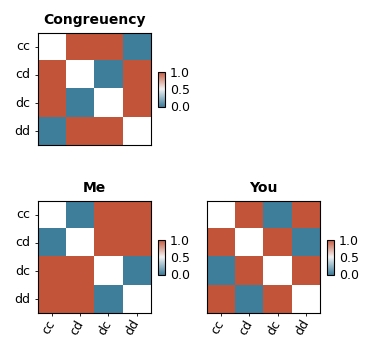
My main hypothesis is that models Me and Congruency should perform similarly well in the two tasks, whereas I expect a difference between the two tasks with respect to model YOU.
My approach at the moment is (1) create RDM_data across time separately for each of the two tasks (using crossnobis), (2) compare the three models to the RDM_data of the two tasks (separately) and finally (3) compare the resulting similarity metrics across the two tasks.
Some papers I have seen use multiple linear regressions for step (2). However, I think I do not have enough data points to correctly perform a linear regression on my observed rdm/models (and I noticed you never mention using it). I have been reading the tutorials and the paper on the use of whitened unbiased measures (Diedrichsen 2021) but unfortunately it is a bit too technical for me to understand which measure is best suited in my case.
I would greatly appreciate any suggestion (including a potential "your whole approach is wrong!"), anything would be very helpful!
Thanks,
Silvia
Beta Was this translation helpful? Give feedback.
All reactions